In recent years, the integration of artificial intelligence (AI) into various aspects of daily life has revolutionized how we interact with technology. One fascinating and increasingly relevant application of AI is in lost pet prediction systems. These systems utilize advanced algorithms to predict where a lost pet might be found based on a variety of factors such as weather conditions, terrain, and even the specific behavior patterns of different breeds. Among these innovative technologies, one particularly interesting case study involves the foggy dog scenario, which highlights the complexities and nuances of predicting a lost pet's location under adverse weather conditions.
The concept of AI-powered lost pet prediction systems is rooted in the ability of machine learning models to analyze vast amounts of data quickly and efficiently. These systems can process information about the pet's breed, age, health status, and previous behavior patterns. By incorporating real-time environmental data, such as temperature, humidity, and visibility, these models can generate predictions that are both accurate and timely. In the context of a foggy dog situation, where visibility is significantly reduced, the system must account for additional variables that could influence the pet's movements.
Foggy conditions present unique challenges for lost pet prediction systems. Fog can obscure landmarks and make it difficult for pets to navigate familiar terrain. This is especially true for dogs, whose reliance on sight can be compromised in such conditions. To address this issue, AI systems incorporate multi-sensor data fusion techniques, combining GPS tracking, infrared sensors, and acoustic signals to enhance the accuracy of their predictions. For instance, if a dog goes missing during a foggy day, the system can use historical data about the dog's past behaviors in similar weather conditions to anticipate its likely path.
One of the key components of these AI systems is the use of neural networks, which are designed to mimic the human brain's ability to learn and adapt. Through deep learning, these networks can identify complex patterns in the data that might not be immediately apparent to human analysts. For example, an AI system might recognize that certain breeds of dogs, like Labradors or Golden Retrievers, tend to seek out open spaces when disoriented by fog, while others, such as terriers, may prefer to stay close to structures or trees. By understanding these behavioral tendencies, the system can provide more precise guidance to pet owners and rescue teams.
Another important aspect of AI-powered lost pet prediction systems is their capacity to integrate social media and community engagement. When a pet goes missing, especially in a foggy environment, time is of the essence. These systems can automatically generate alerts on platforms like Facebook, Twitter, and Nextdoor, providing detailed descriptions of the pet and its potential whereabouts. Additionally, they can leverage crowdsourced data from local residents who may have seen the pet, further refining the prediction model in real-time.
The foggy dog scenario also underscores the importance of continuous improvement in AI systems. As more data is collected over time, the algorithms become better at predicting outcomes under varying conditions. Machine learning models are trained using supervised and unsupervised learning techniques, allowing them to improve their accuracy without explicit programming. This iterative process ensures that the system remains effective even as new challenges, such as changing weather patterns or urban development, emerge.
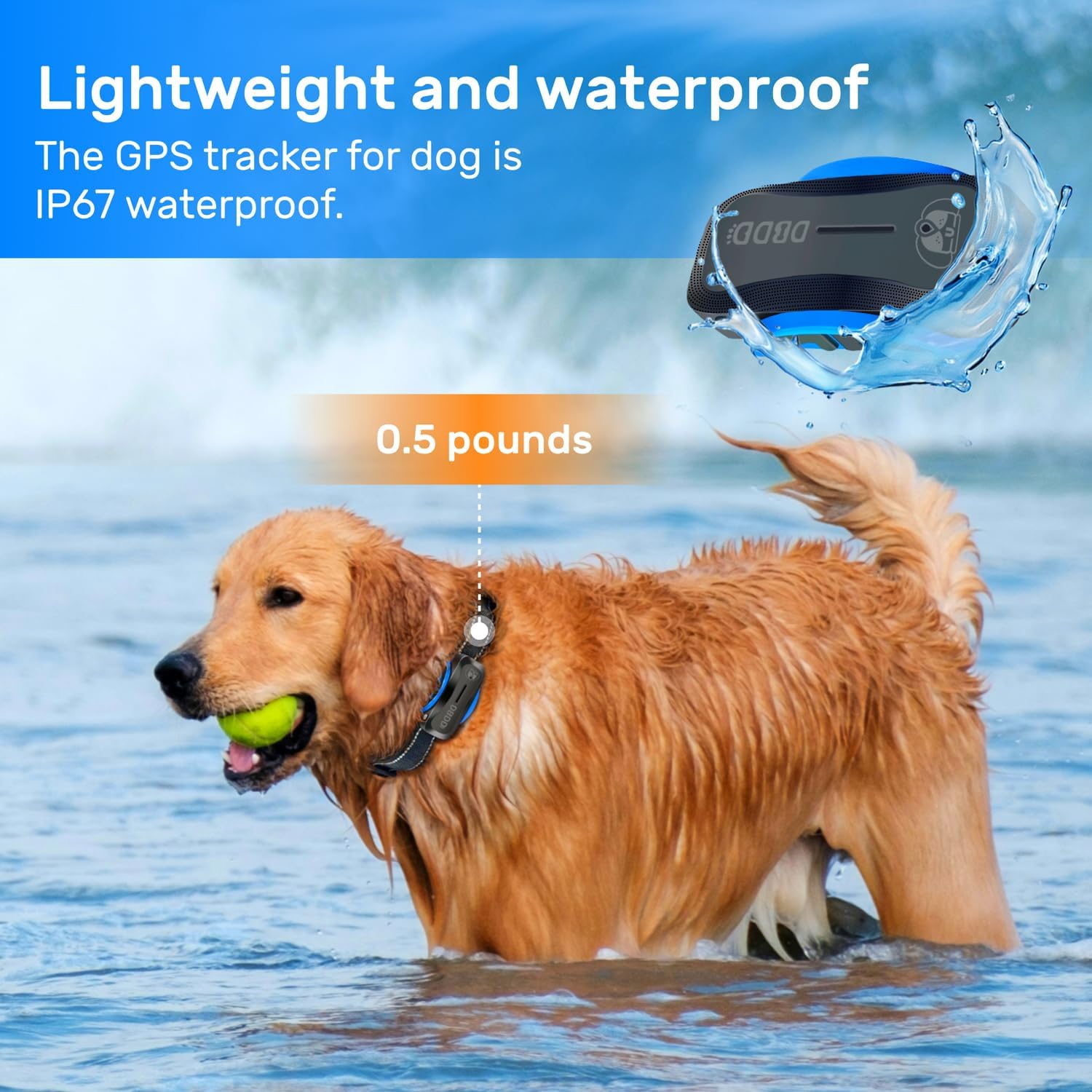
Moreover, AI-powered lost pet prediction systems often include features that allow for personalized recommendations based on individual pet profiles. Owners can input detailed information about their pets, including temperament, favorite hiding spots, and known triggers for wandering. This data is then used to tailor the prediction model specifically to that pet, increasing the likelihood of a successful recovery. For example, if a particular dog has a history of becoming anxious in foggy conditions and tends to retreat to a nearby park, the system can prioritize that area in its search recommendations.
Despite the advancements in AI technology, there are still limitations and challenges to overcome. One major concern is the reliability of the data being fed into the system. If the input data is incomplete or inaccurate, the predictions generated by the system may not be trustworthy. Additionally, privacy issues arise when collecting and sharing sensitive information about pets and their owners. Developers must ensure that proper safeguards are in place to protect this data and maintain user trust.
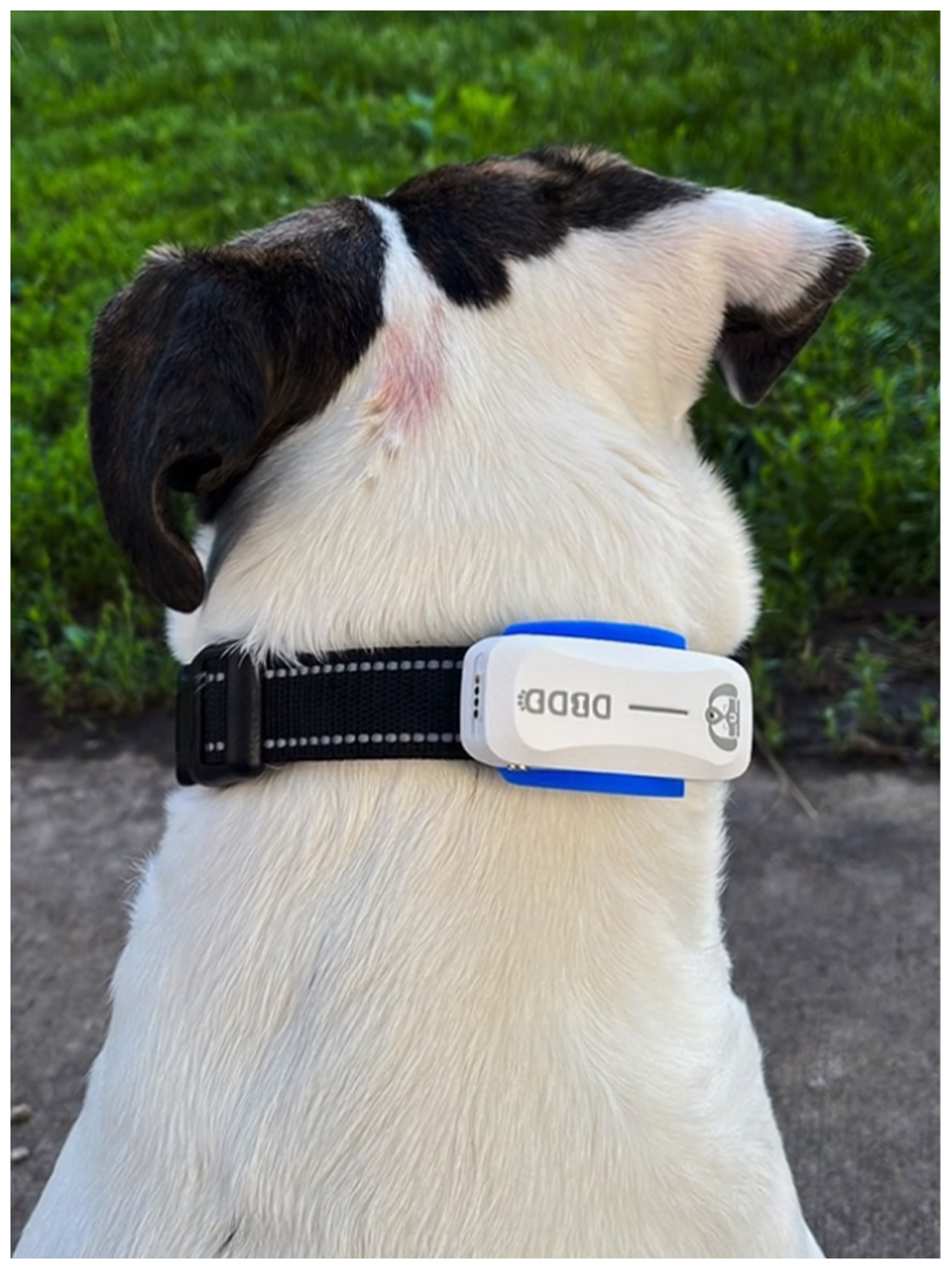
Furthermore, the effectiveness of AI-powered lost pet prediction systems depends heavily on the cooperation between technology providers, animal welfare organizations, and local communities. Collaboration is essential to maximize the reach and impact of these systems. For instance, partnerships with shelters and rescue groups can help disseminate information more widely and provide resources for recovering lost pets. Similarly, involving community members in the process can lead to faster identifications and recoveries, as more eyes are searching for the missing animals.
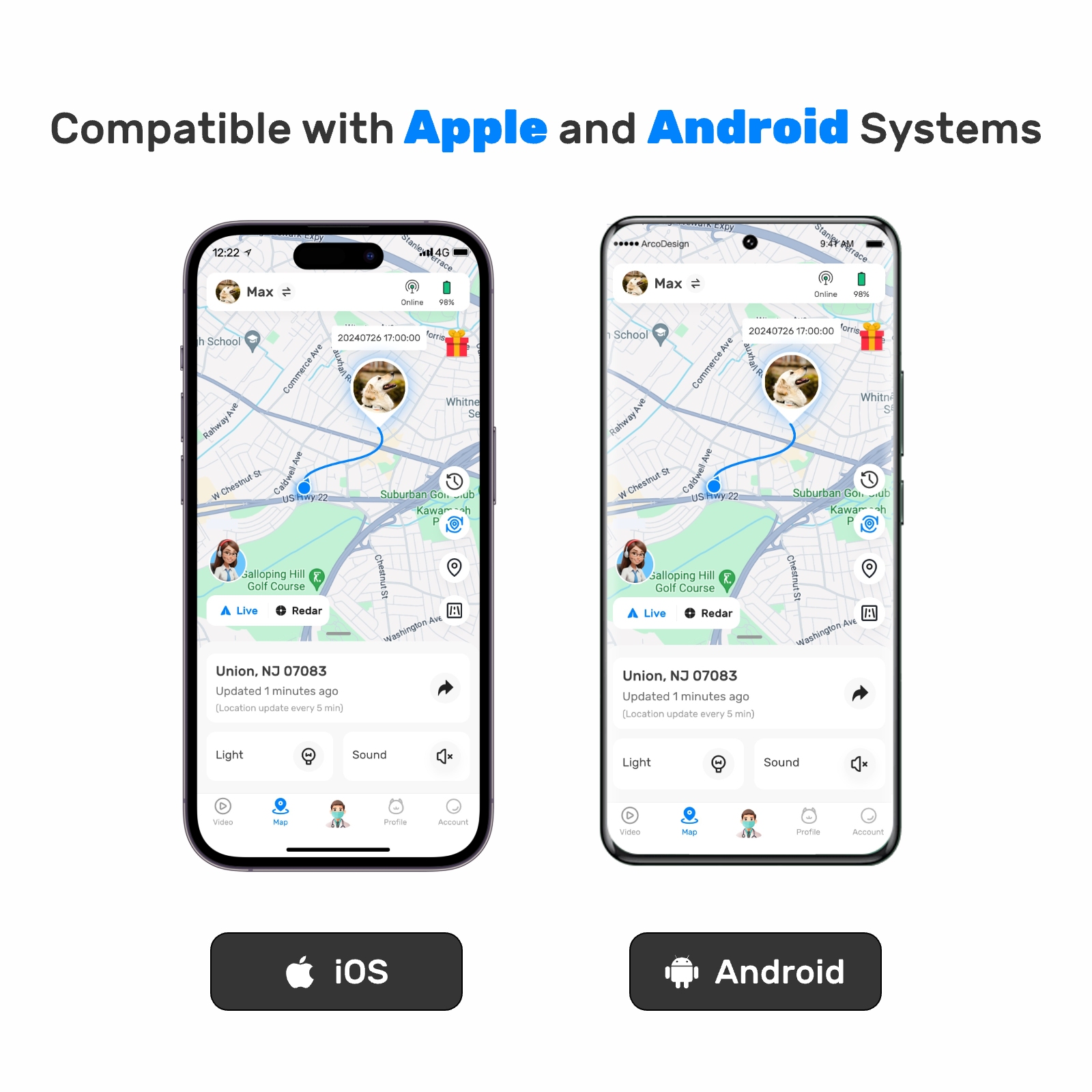
Looking ahead, the future of AI-powered lost pet prediction systems holds great promise. As technology continues to evolve, we can expect even more sophisticated models that incorporate emerging innovations such as quantum computing and edge AI. These advancements will enable faster processing speeds and greater computational power, allowing for real-time analysis of vast datasets. Additionally, the integration of autonomous drones equipped with AI capabilities could revolutionize search and rescue operations, particularly in challenging environments like foggy forests or urban jungles.
In conclusion, AI-powered lost pet prediction systems represent a significant leap forward in our ability to locate and recover missing animals. The foggy dog scenario exemplifies the complexity of this task and the necessity for robust, adaptable solutions. By leveraging advanced algorithms, multi-sensor data fusion, and community engagement, these systems offer hope to countless pet owners whose furry companions have wandered off. As we continue to refine and expand these technologies, the ultimate goal remains clear: to bring every lost pet safely back home.
Update Time:2025-05-15 07:45:57