Lost pets are a common concern for many pet owners, causing stress and worry as they search for their beloved companions. The emergence of AI-powered systems has introduced new possibilities in addressing this issue, including the development of lost pet prediction systems. These systems utilize advanced algorithms to predict where a lost pet might be found based on various data points, such as past behavior patterns, environmental conditions, and geographical information. Among these systems, one particularly fascinating application involves straw dogs, which have been used historically as symbolic representations of domestic canines but now serve as a unique model for understanding pet movement patterns.
The concept of using AI to predict where lost pets might be found is rooted in the ability to analyze large datasets quickly and efficiently. By collecting data from GPS trackers, social media posts, and local animal shelters, these systems create predictive models that estimate the most likely locations where a missing pet could be located. Straw dogs, though not real animals, provide an interesting framework for testing these models because their movements can be simulated under controlled conditions. This allows researchers to refine their algorithms without needing to rely solely on actual lost pet scenarios, which can be unpredictable and emotionally charged.
Straw dogs, traditionally used in cultural rituals or as decorative items, represent an idealized version of canine behavior. When incorporated into AI models, they help simulate different types of dog behaviors—ranging from adventurous wanderers to more homebound pets. By programming virtual straw dogs with varying tendencies, researchers can test how accurately their prediction systems perform across diverse scenarios. For instance, a straw dog programmed to mimic a highly active breed like a Border Collie would move differently than one representing a less energetic breed like a Bulldog. These simulations allow for the optimization of prediction algorithms by accounting for differences in activity levels, age, and even temperament.
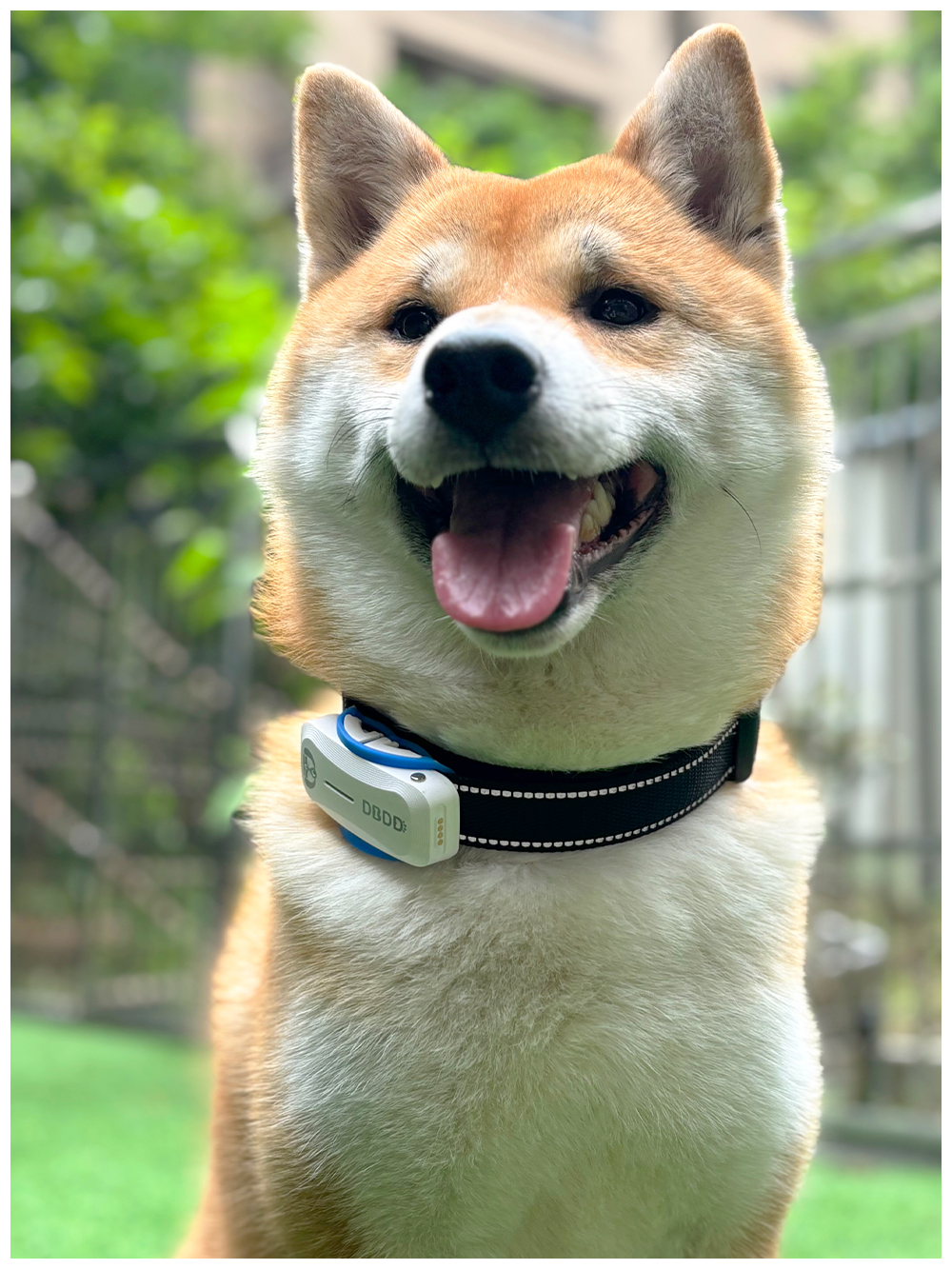
Moreover, the integration of machine learning techniques enhances the effectiveness of these prediction systems. Machine learning enables the system to learn from previous cases of lost pets, improving its accuracy over time. As it processes more data, the system becomes better at identifying patterns and making predictions about future incidents. For example, if certain breeds tend to travel farther when lost compared to others, the system will adjust its parameters accordingly. Similarly, environmental factors such as weather conditions, terrain type, and proximity to roads or water bodies also influence a pet's movement. By incorporating these variables into the model, the system gains a comprehensive understanding of potential outcomes.
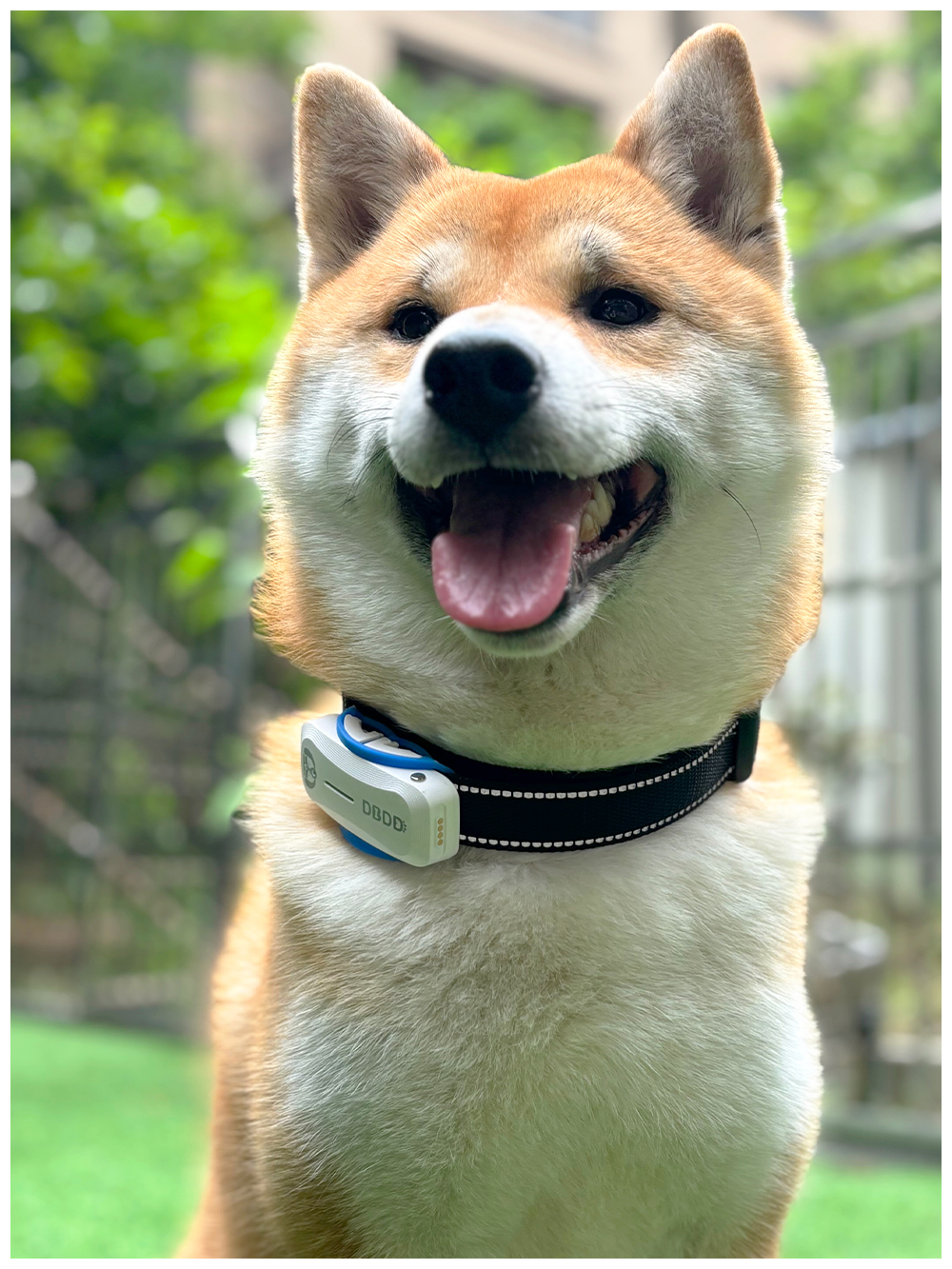
Another significant advantage of AI-powered lost pet prediction systems is their ability to leverage community participation. Many platforms encourage users to report sightings of lost pets through mobile apps or websites. These reports contribute valuable real-time data that the system uses to update its predictions dynamically. In some cases, citizens may use augmented reality (AR) features to capture images of stray animals, which are then analyzed by the AI to determine whether they match descriptions of missing pets. This collaborative approach not only increases the chances of reuniting pets with their owners but also fosters a sense of community involvement.
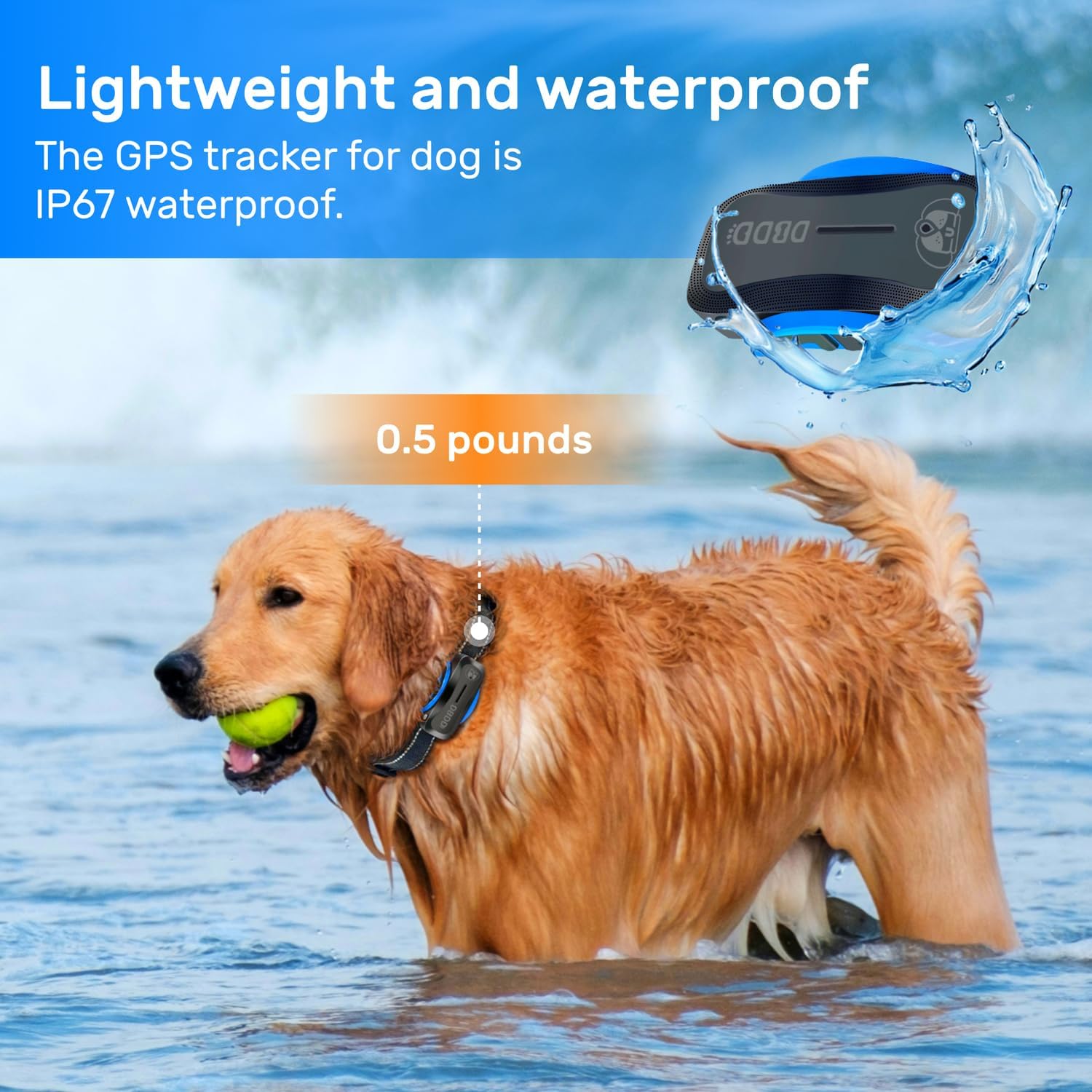
The role of straw dogs in this context extends beyond mere simulation. They symbolize the importance of considering both practical and theoretical aspects when developing predictive technologies. Just as straw dogs were once crafted to serve specific purposes in ancient traditions, modern AI systems must be designed thoughtfully to address the needs of pet owners while respecting ethical considerations. Privacy concerns arise when tracking pets' movements, especially since the same technology could theoretically be applied to humans. Therefore, developers must ensure that their systems prioritize user consent and data protection.
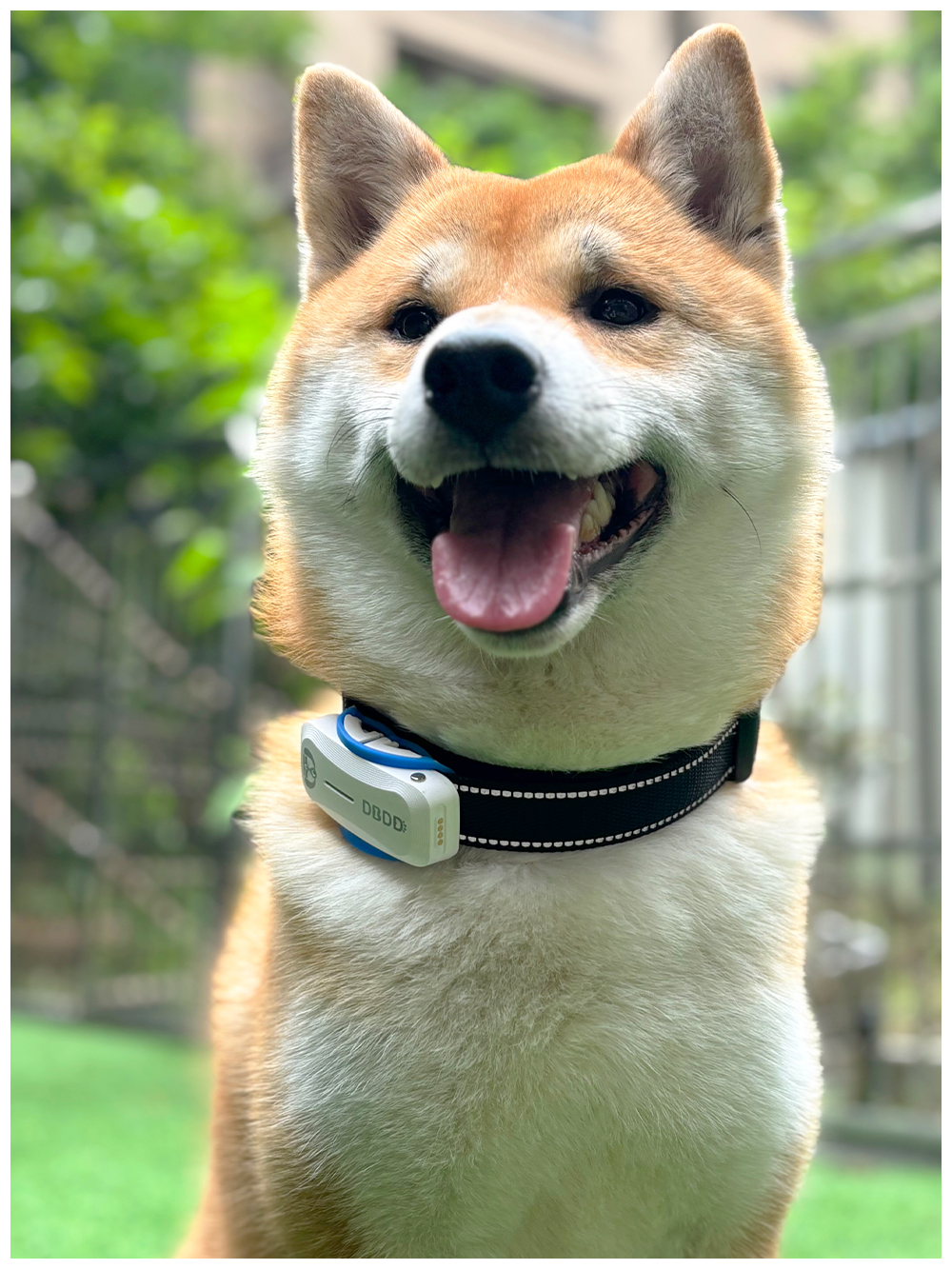
Additionally, the use of straw dogs highlights the value of interdisciplinary approaches in technological innovation. Historians, anthropologists, and sociologists can offer insights into the symbolic meanings associated with straw dogs, helping technologists design solutions that resonate culturally as well as functionally. For instance, understanding the historical significance of straw dogs in various cultures might inspire creative ways to engage communities in efforts to locate lost pets. Perhaps promotional campaigns featuring straw dog imagery could encourage greater participation among diverse populations.
Despite the promise of AI-powered lost pet prediction systems, challenges remain. One major hurdle is ensuring the reliability of predictions given the unpredictability inherent in animal behavior. While simulations involving straw dogs provide useful benchmarks, no algorithm can fully account for every variable affecting a real pet's journey after becoming lost. Factors such as fear responses, hunger, thirst, and interactions with other animals introduce complications that even the most sophisticated models cannot always anticipate. Consequently, developers must continuously improve their systems to handle unexpected situations effectively.
Furthermore, accessibility issues need addressing. Not all pet owners have access to the latest technology or the financial means to invest in high-end tracking devices. To maximize impact, designers should strive to create inclusive solutions that benefit underserved communities. Open-source tools and affordable hardware options could democratize access to these life-saving innovations. Education initiatives teaching people how to use these resources responsibly would further enhance their utility.
Incorporating feedback loops into the system design represents another critical aspect of ongoing improvement. Pet owners who successfully recover their animals using the prediction system should be encouraged to share their experiences so developers can fine-tune the algorithms. Anonymous surveys or direct communication channels facilitate this process, allowing for continuous refinement based on real-world results rather than hypothetical assumptions alone.
Looking ahead, the convergence of AI, IoT (Internet of Things), and blockchain technologies holds exciting possibilities for enhancing lost pet prediction systems. Imagine a network where each registered pet carries a lightweight wearable device connected to a decentralized ledger. This setup would enable secure sharing of location data while maintaining privacy safeguards. Smart contracts could automate reward distributions for individuals who assist in finding lost pets, incentivizing broader participation.
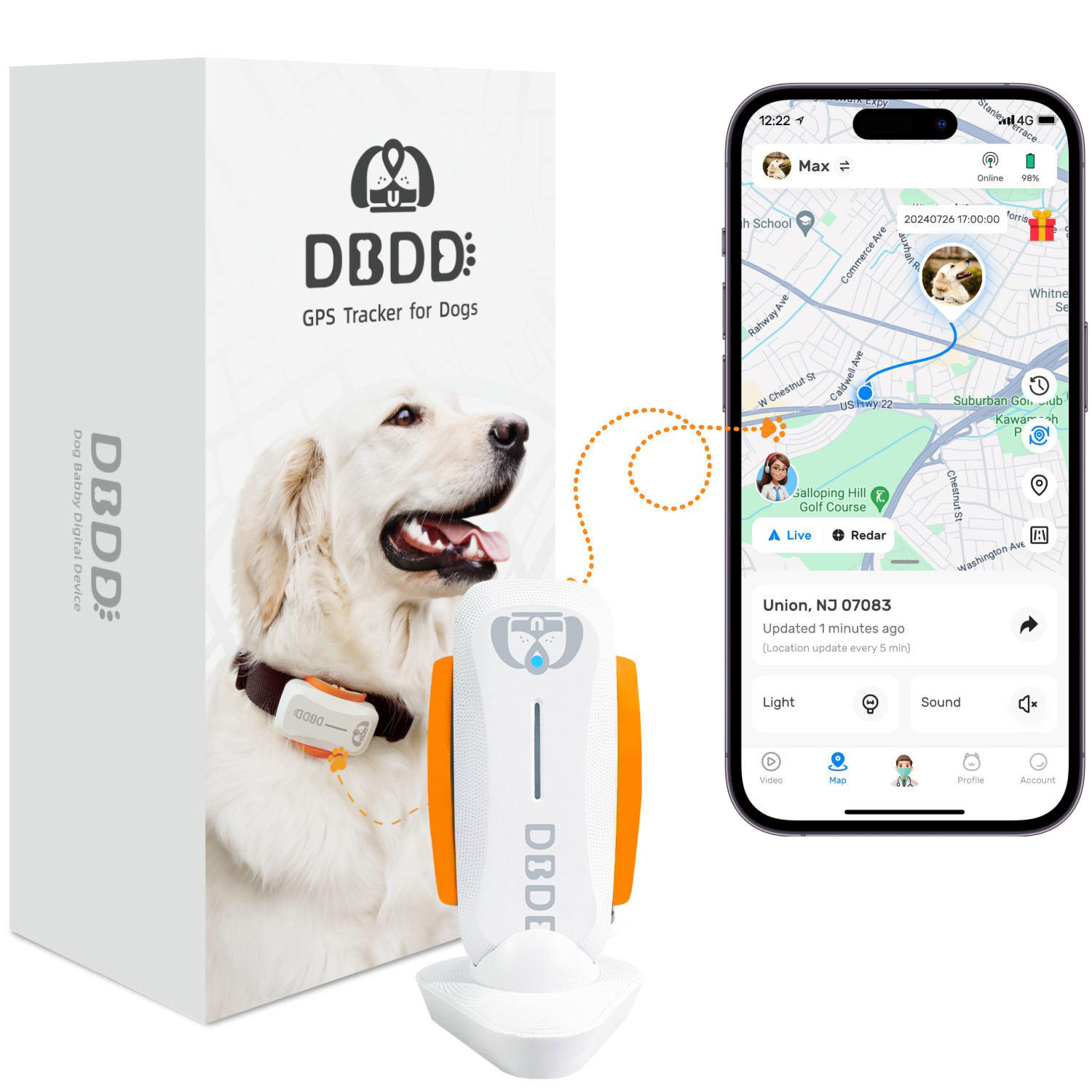
Returning to the theme of straw dogs, we see parallels between their traditional roles and the aspirations of contemporary AI developers. Both aim to bridge gaps—whether spiritual or informational—by creating representations that embody desired qualities or outcomes. In the case of AI-powered lost pet prediction systems, those qualities include precision, empathy, and adaptability. By drawing inspiration from symbols like straw dogs, innovators remind themselves of the deeper connections linking humans, animals, and technology throughout history.
Ultimately, the success of these systems depends not only on technical prowess but also on fostering trust between creators and users. Transparent communication regarding capabilities, limitations, and ethical guidelines builds confidence in the technology. Encouraging open dialogue between stakeholders ensures that evolving demands and expectations shape future iterations positively.
As society continues advancing technologically, opportunities abound for applying artificial intelligence toward solving everyday problems creatively. Lost pet prediction systems exemplify this trend, demonstrating how seemingly disparate elements—like straw dogs and machine learning—can combine synergistically to produce meaningful benefits. Whether through simulating idealized behaviors or facilitating community collaboration, these innovations underscore the transformative potential of thoughtful integration across disciplines.
Update Time:2025-05-15 07:11:34