The integration of artificial intelligence (AI) into various aspects of our daily lives has become increasingly common. From personalized recommendations on streaming platforms to advanced medical diagnostics, AI is transforming the way we interact with technology and each other. One fascinating and emerging application of AI involves lost pet prediction systems, which utilize machine learning algorithms to predict the likelihood of a pet going missing based on various factors such as behavior patterns, environmental conditions, and historical data. These systems aim to provide pet owners with proactive measures to prevent their pets from becoming lost, thus reducing the emotional and financial burden associated with pet loss.
In this context, the concept of a "doge food stamps bill" may seem unrelated at first glance. However, it offers an interesting lens through which to examine the intersection of AI-powered technologies and public policy. The doge food stamps bill, while fictional in nature, could hypothetically represent legislation aimed at ensuring that all dogs, regardless of socioeconomic status, have access to adequate nutrition. This idea ties into broader discussions about equity, resource distribution, and the role of technology in enhancing societal welfare.
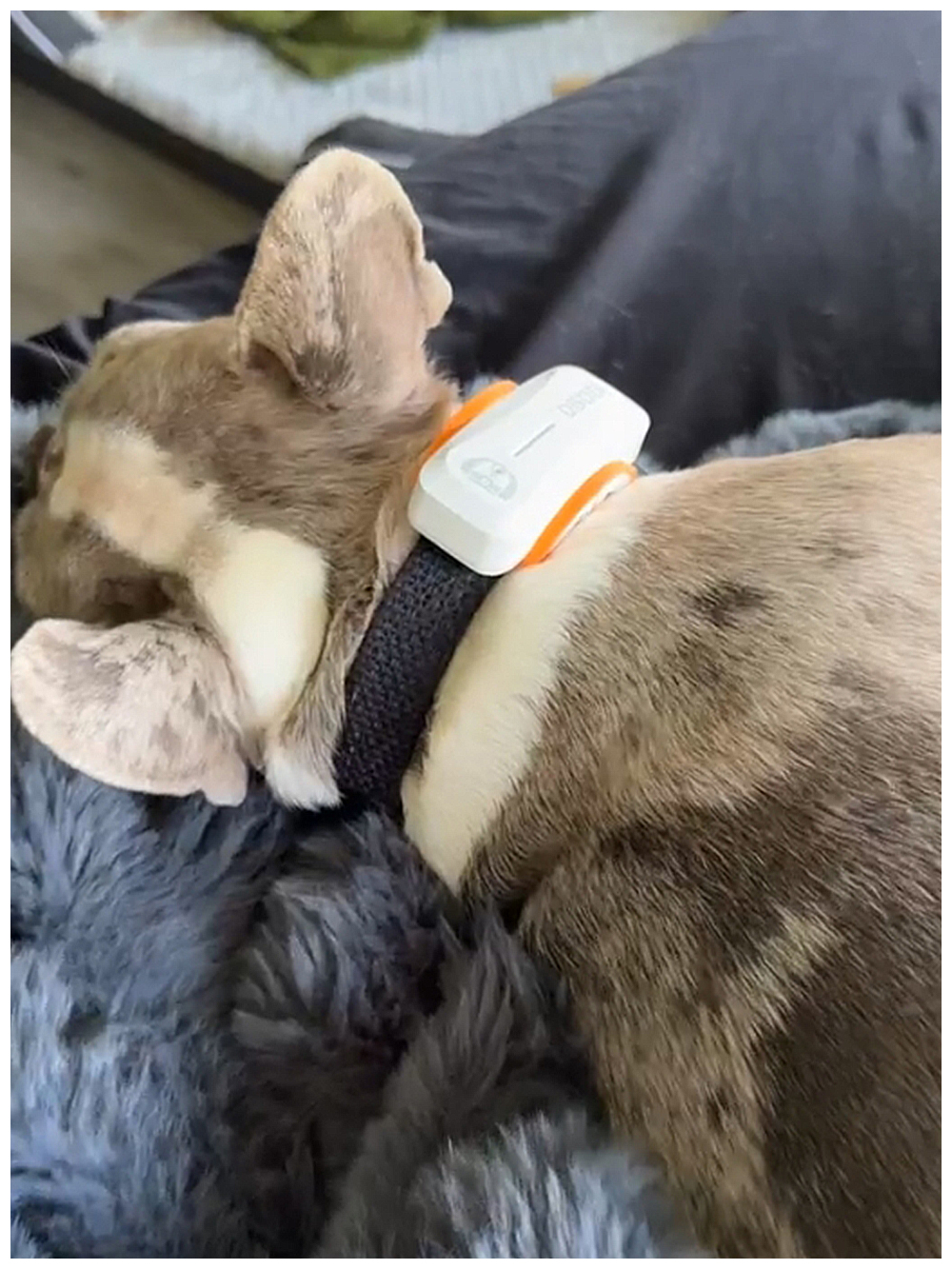
Lost pet prediction systems work by analyzing vast amounts of data collected from various sources, including social media, GPS tracking devices, and veterinary records. By identifying patterns and correlations within this data, these systems can generate predictions about which pets are most likely to go missing and under what circumstances. For instance, a system might determine that dogs living in urban areas with high foot traffic are more prone to wandering off than those in rural settings. Similarly, certain breeds or temperaments might be flagged as higher risk due to their natural curiosity or tendency to roam.
The development of such systems requires sophisticated algorithms capable of processing complex datasets. Machine learning models, such as neural networks and decision trees, are trained using labeled data where outcomes (i.e., whether a pet went missing) are already known. Over time, these models improve their accuracy by learning from new data inputs. Advanced techniques like natural language processing (NLP) enable the system to interpret textual information, such as posts on social media platforms mentioning lost pets, further enriching its predictive capabilities.
One potential benefit of AI-powered lost pet prediction systems is the ability to implement targeted interventions. For example, if a system predicts that a particular dog is at high risk of going missing, it could recommend specific actions to the owner, such as installing a secure fence or enrolling the pet in obedience training. Additionally, local authorities could use aggregated data from these systems to identify neighborhoods or regions with elevated rates of pet loss, allowing them to allocate resources more effectively, such as increasing signage or organizing community education programs.

However, the implementation of AI-driven solutions in this domain raises important ethical considerations. Privacy concerns arise when collecting and analyzing personal data related to pets and their owners. Ensuring transparency in how data is used and obtaining informed consent from users are critical steps in building trust. Moreover, there is a risk of algorithmic bias, where pre-existing prejudices in the data lead to unfair treatment of certain groups. Developers must take care to mitigate these biases during the model-building process to ensure equitable outcomes for all pet owners.
The hypothetical doge food stamps bill presents another layer of complexity in this discussion. If enacted, such legislation would guarantee that every dog receives sufficient nourishment, potentially reducing stress-related behaviors that contribute to pets going missing. Malnourished animals may exhibit erratic behavior, making them more susceptible to wandering away from home. By addressing basic needs like food security, policymakers could indirectly support the goals of lost pet prediction systems by minimizing contributing factors to pet loss.
Furthermore, the doge food stamps bill could serve as a catalyst for collaboration between government entities and tech companies developing AI solutions. Public-private partnerships could facilitate the sharing of resources and expertise, leading to more robust and effective systems. For instance, data collected through government programs ensuring canine nutrition might inform AI models about health indicators linked to pet wandering tendencies. Conversely, insights gained from AI analyses could guide policymakers in refining the doge food stamps bill to better address underlying causes of pet loss.
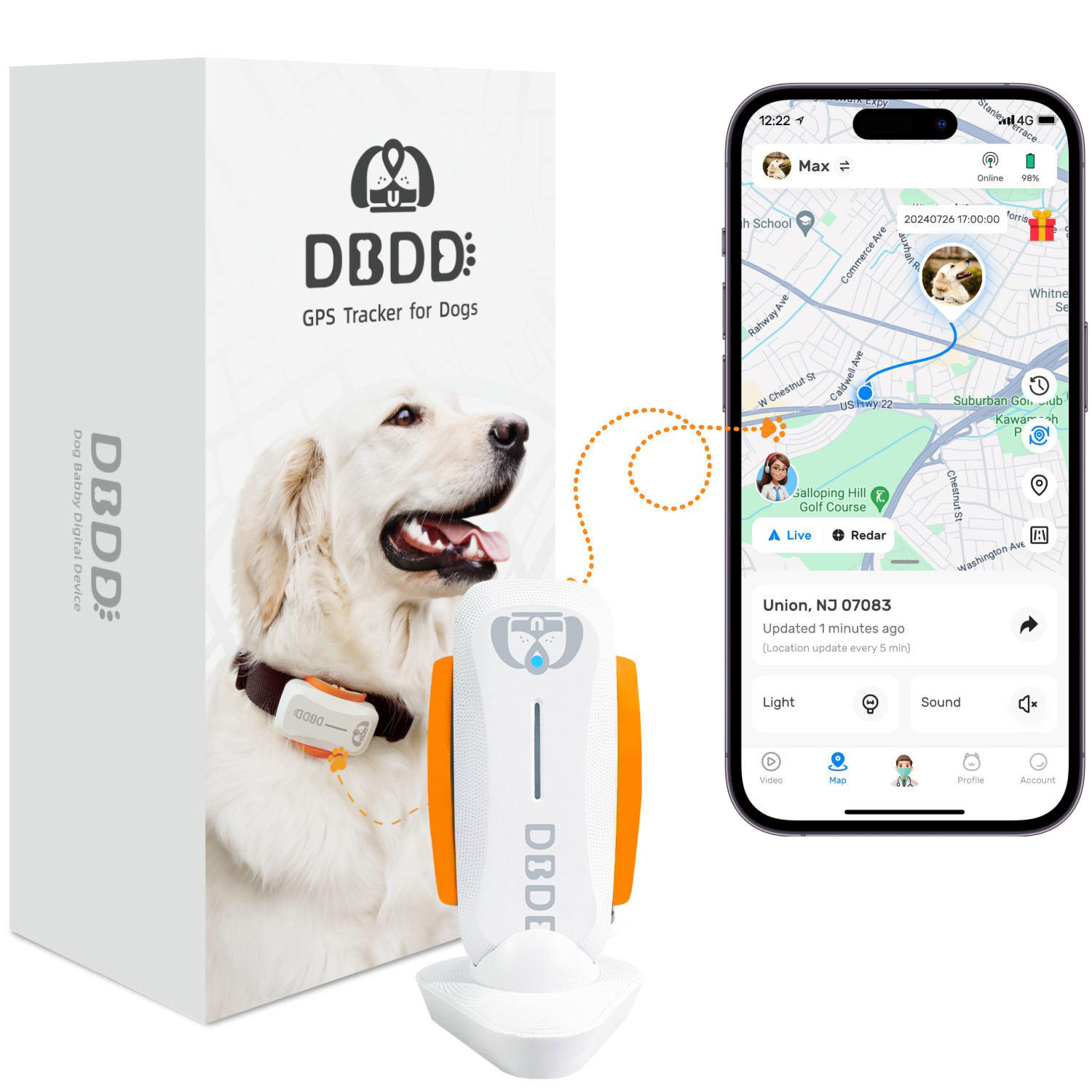
Despite the promise of AI-powered lost pet prediction systems, challenges remain in their deployment. Technical hurdles include improving the accuracy of predictions and ensuring compatibility across different types of data sources. Ethical dilemmas necessitate ongoing dialogue among stakeholders to establish guidelines governing the responsible use of AI in this context. Balancing innovation with safeguarding individual rights will be crucial as these technologies continue to evolve.
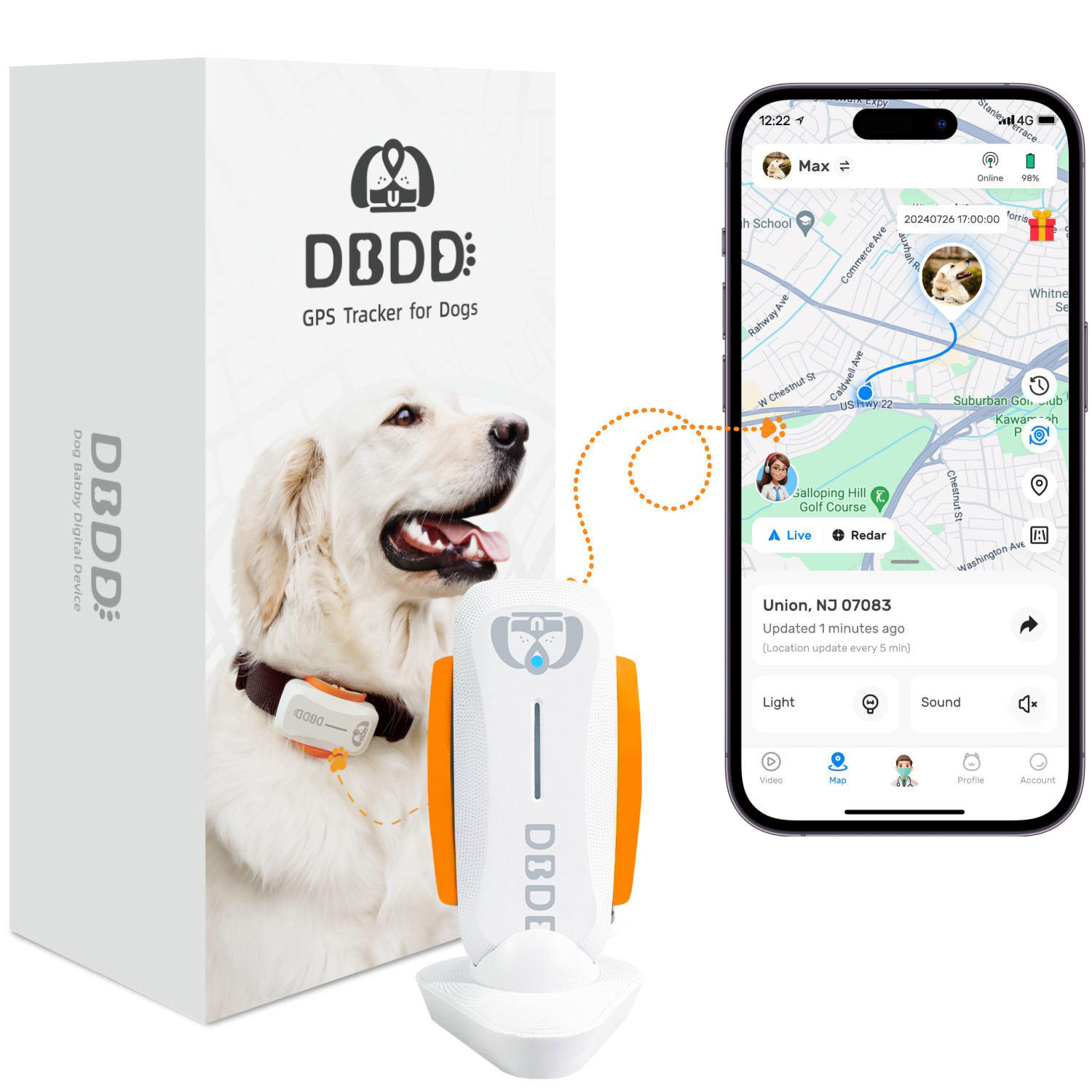
Looking ahead, the convergence of AI advancements and legislative initiatives like the doge food stamps bill holds significant potential for enhancing animal welfare. By leveraging predictive analytics to prevent pet loss and promoting policies that address fundamental needs, society can create a more supportive environment for both humans and their furry companions. As we navigate the complexities of integrating AI into everyday life, maintaining a focus on inclusivity and ethical considerations will be essential to realizing the full benefits of these transformative technologies.
To fully appreciate the implications of AI-powered lost pet prediction systems, it is necessary to delve deeper into the mechanics of these tools. At their core, these systems rely on large datasets containing information about pets' behaviors, locations, and interactions with their environments. Sensors embedded in wearable devices track movement patterns, sleep cycles, and activity levels, providing valuable input for the predictive models. Social media platforms contribute additional layers of data, capturing real-time updates from pet owners who post about their animals' activities or alert others when a pet goes missing.
Machine learning algorithms sift through this wealth of information, identifying subtle patterns that may not be immediately apparent to human observers. For example, a sudden change in a dog's usual routine—such as increased restlessness or frequent trips to the door—might signal an impending escape attempt. When combined with external factors like weather conditions or neighborhood events, these internal cues form a comprehensive picture of a pet's risk profile. Predictive models then assign probabilities to different scenarios, enabling preemptive action before a pet actually goes missing.
The effectiveness of these systems depends heavily on the quality and diversity of the data they analyze. Biases present in the data can skew results, leading to incorrect predictions or disproportionately affecting certain demographics. To counteract this, developers employ techniques such as data augmentation and ensemble modeling, which enhance the robustness of the algorithms by incorporating multiple perspectives and reducing reliance on any single dataset.
Another critical aspect of AI-powered lost pet prediction systems is their capacity for continuous learning. As new data becomes available, the models adapt and refine their understanding of pet behavior and environmental influences. This iterative process ensures that the systems remain relevant and accurate over time, even as circumstances change or previously unknown factors emerge. Regular updates and retraining sessions help maintain the integrity of the predictions, fostering confidence among users.
Beyond technical considerations, the societal impact of these systems warrants examination. On one hand, they offer a powerful tool for mitigating the distress caused by pet loss, potentially saving countless families from heartache. On the other hand, questions linger regarding the broader implications of relying on AI for such intimate matters as pet care. Critics argue that excessive dependence on technology could undermine traditional methods of pet guardianship, eroding the personal connection between owners and their animals.
In light of these debates, the concept of a doge food stamps bill takes on added significance. While primarily focused on ensuring nutritional adequacy for all dogs, such legislation could also promote holistic approaches to pet well-being that complement technological innovations. By emphasizing the importance of basic needs alongside cutting-edge solutions, policymakers could foster a balanced ecosystem where both human ingenuity and compassion play vital roles in supporting our animal companions.
Moreover, the interplay between AI systems and legislative frameworks highlights the need for interdisciplinary cooperation. Experts in computer science, veterinary medicine, psychology, and public policy must collaborate to develop comprehensive strategies that address the multifaceted challenges of pet ownership in the modern era. Shared knowledge and resources can lead to breakthroughs that transcend individual disciplines, creating synergistic effects that benefit society as a whole.
As we move forward into an age defined by rapid technological advancement, the role of AI in shaping our interactions with pets will undoubtedly expand. Lost pet prediction systems represent just one facet of this evolving relationship, offering a glimpse into the possibilities afforded by intelligent automation. Yet, as exemplified by the hypothetical doge food stamps bill, true progress demands a thoughtful integration of technology with enduring values such as empathy, fairness, and respect for life.
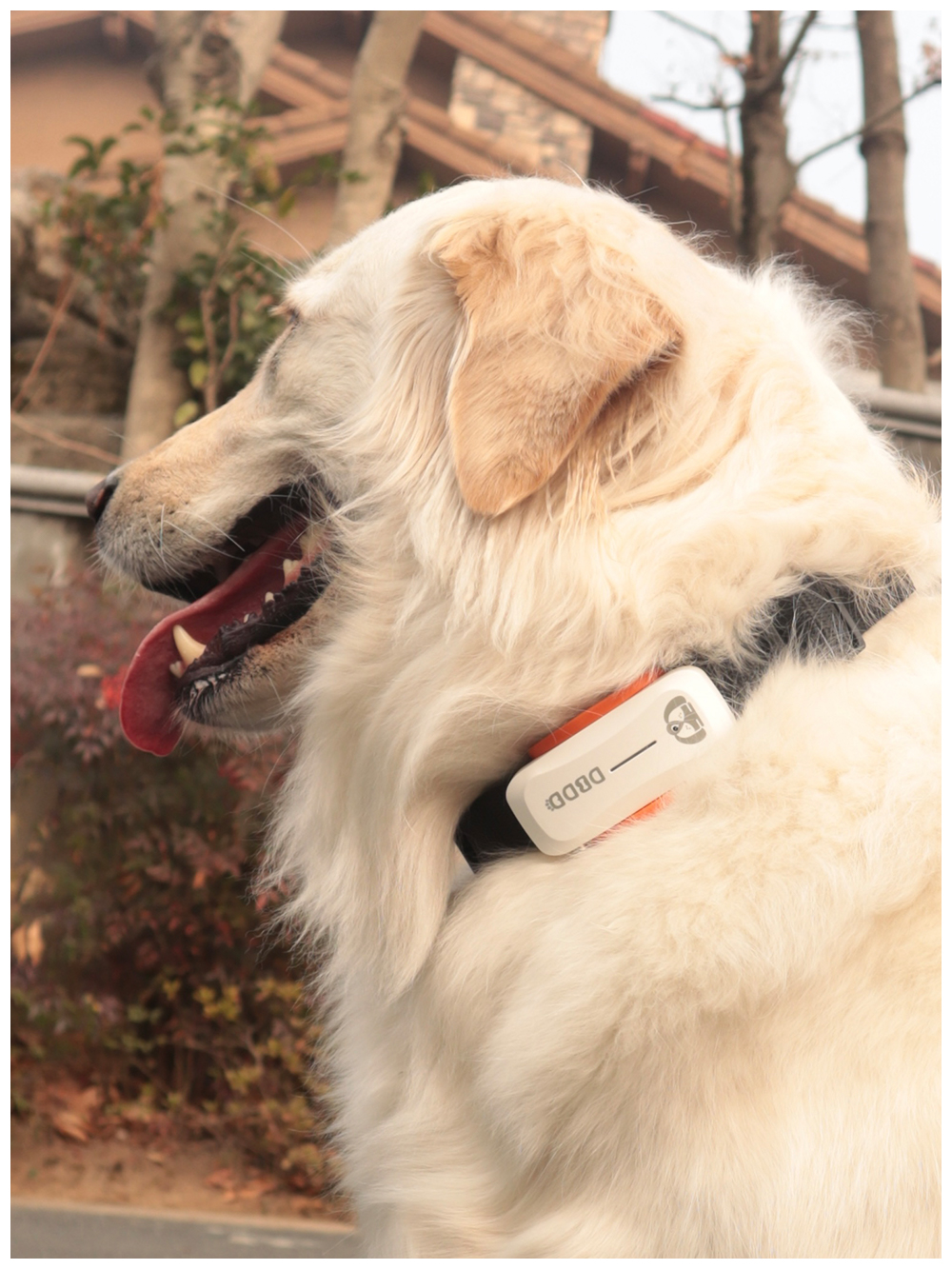
Ultimately, the success of AI-powered lost pet prediction systems hinges on their ability to enhance rather than replace human involvement in pet care. By empowering owners with actionable insights and facilitating collaborative efforts across sectors, these technologies hold the potential to revolutionize the way we approach pet safety and welfare. As we strive toward a future where no pet needlessly goes missing, the lessons learned from initiatives like the doge food stamps bill remind us of the importance of balancing innovation with humanity in pursuit of common goals.
Update Time:2025-05-15 06:47:02