Losing a pet can be one of the most distressing experiences for any pet owner. The anxiety and uncertainty that accompany such situations often leave owners scrambling to find ways to locate their beloved companions quickly. In recent years, advancements in artificial intelligence (AI) have opened up new possibilities for addressing this issue. One particularly innovative approach involves AI-powered lost pet prediction systems that analyze dog barking sound patterns. This technology leverages machine learning algorithms to interpret the unique vocalizations of dogs, helping predict when a pet might wander off or become lost. This article delves into the intricacies of these systems, exploring how they work, their potential applications, and the challenges they face.
At the heart of these AI-powered systems is the ability to recognize and interpret various types of dog barking sound. Dogs communicate through a variety of vocalizations, each with distinct meanings. For instance, a bark may indicate excitement, fear, aggression, or even loneliness. By analyzing these sounds, AI systems can gain insights into a dog's emotional state and behavior patterns. This capability is crucial because changes in a dog's barking habits could signal an increased likelihood of wandering or becoming lost. For example, if a dog starts barking more frequently due to boredom or loneliness, it might be more inclined to escape its confines in search of stimulation or companionship.
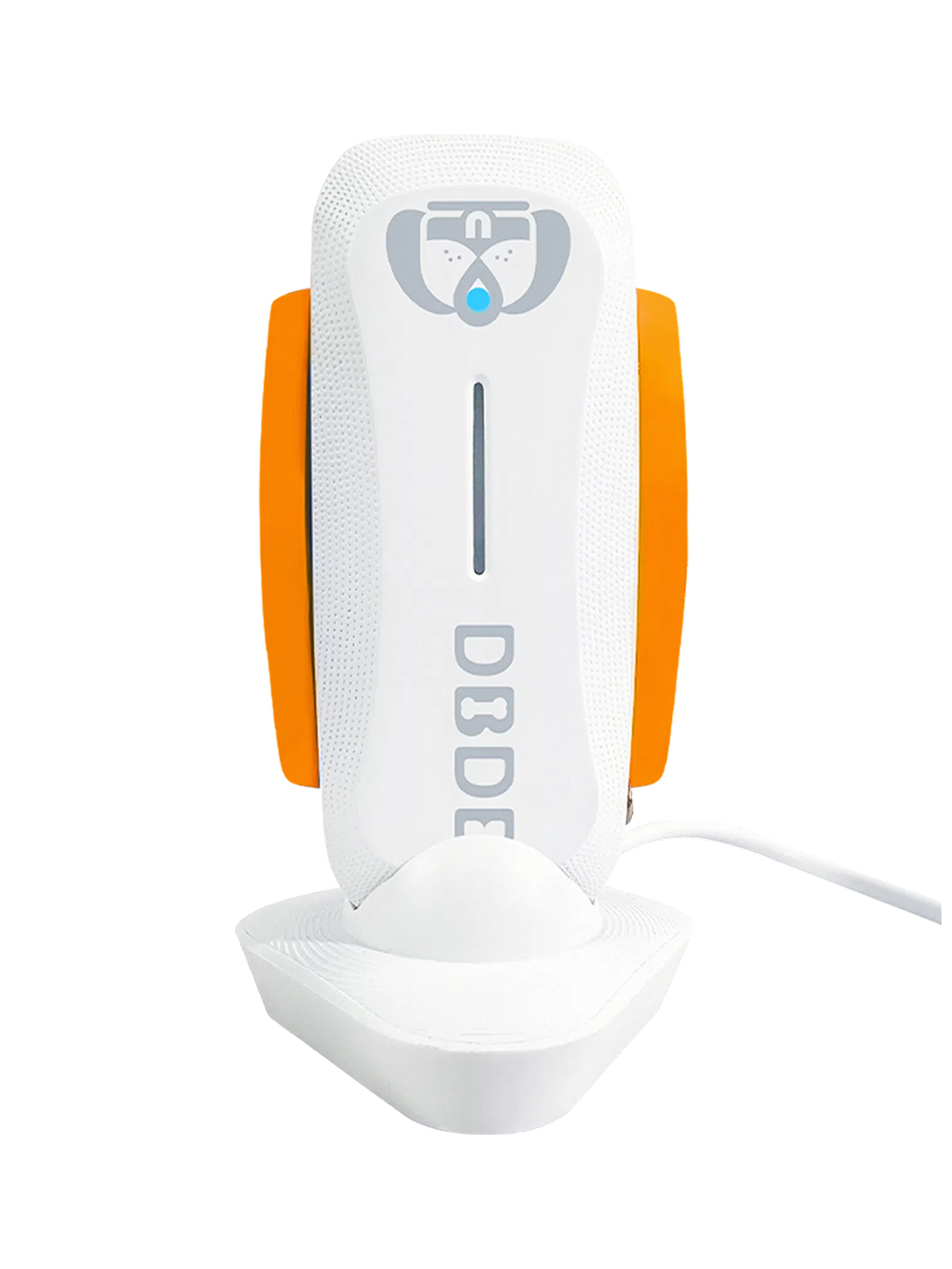
The process begins with data collection. Modern wearable devices for pets, equipped with microphones and other sensors, allow for continuous monitoring of a dog's activities and vocalizations. These devices capture the dog barking sound in real-time, storing the data for later analysis. The collected audio is then processed using advanced signal processing techniques to isolate the barks from other ambient noises. Once the barks are identified, they are fed into machine learning models specifically trained to recognize different types of barking and associate them with particular behaviors or emotional states.
Machine learning plays a pivotal role in making sense of the vast amount of data generated by these devices. Algorithms such as neural networks are employed to learn patterns in the data over time. Through supervised learning, where the system is trained on labeled datasets, the AI can classify barks based on their characteristics, such as pitch, duration, and frequency. Unsupervised learning methods, on the other hand, enable the discovery of hidden patterns without prior labeling, allowing the system to adapt to new and unexpected barking patterns.
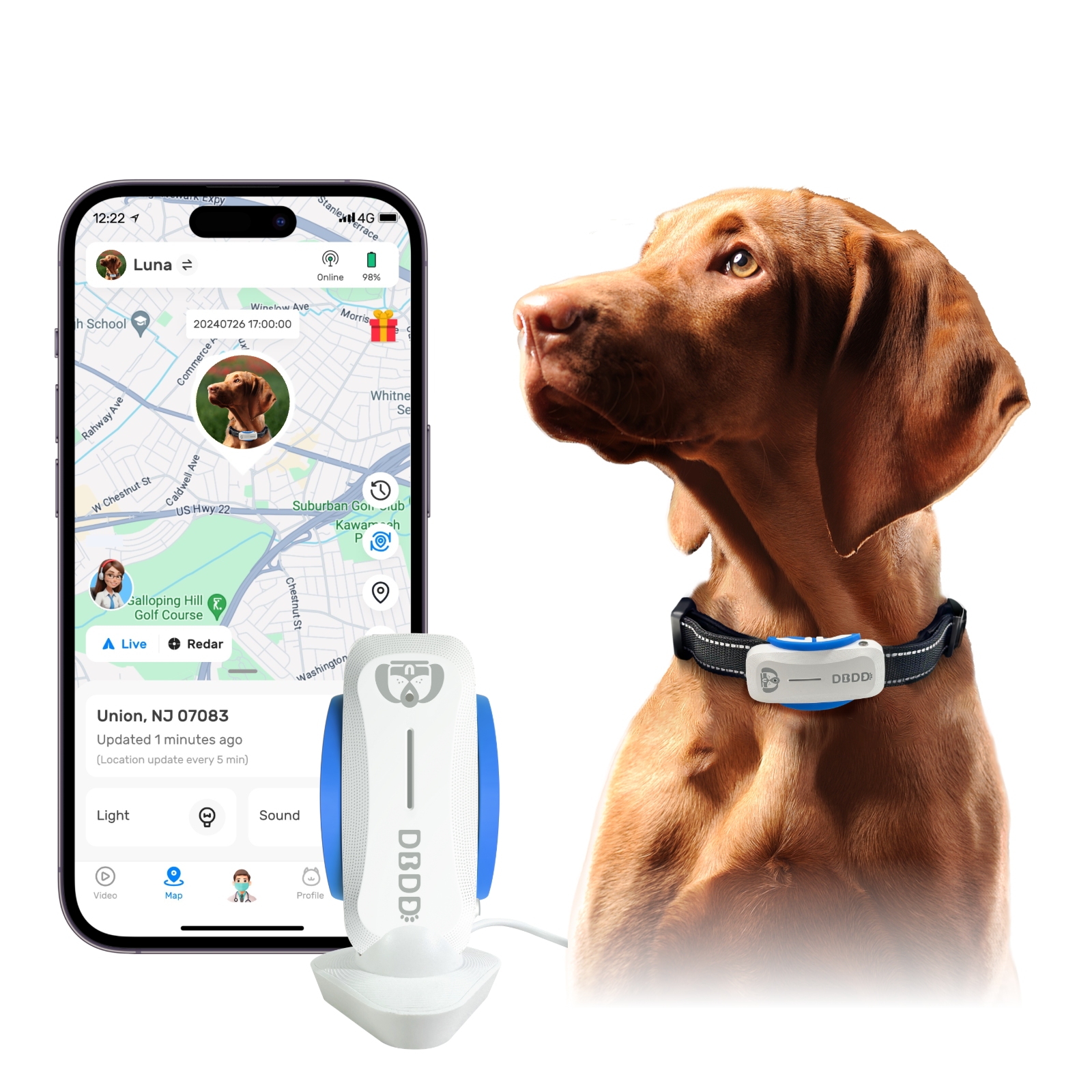
One of the key advantages of AI-powered lost pet prediction systems is their ability to provide early warnings. By continuously monitoring a dog's barking sound, these systems can detect anomalies that might indicate an impending escape attempt. For example, if a normally quiet dog suddenly starts barking excessively at night, the system could alert the owner to check on the dog and ensure it is secure. Similarly, if a dog exhibits a pattern of barking associated with frustration or agitation, the system might suggest interventions to address the underlying cause, such as providing more exercise or mental stimulation.
Moreover, these systems can integrate additional data sources to enhance their predictive capabilities. GPS tracking data, for instance, can provide context about a dog's location and movements, helping to confirm whether a dog has indeed wandered off or is merely expressing itself vocally. Environmental sensors can also contribute valuable information, such as weather conditions or nearby disturbances, which might influence a dog's behavior. By combining multiple data streams, the AI system gains a more comprehensive understanding of the dog's situation, leading to more accurate predictions.
Despite their promise, AI-powered lost pet prediction systems face several challenges that need to be addressed for widespread adoption. One major hurdle is ensuring the accuracy and reliability of the predictions. False positives, where the system incorrectly predicts that a dog will become lost, can lead to unnecessary stress for pet owners. Conversely, false negatives, where the system fails to predict an actual escape, could result in lost opportunities to prevent the incident. To mitigate these risks, developers must continually refine the algorithms and expand the training datasets to encompass a broader range of scenarios and breeds.
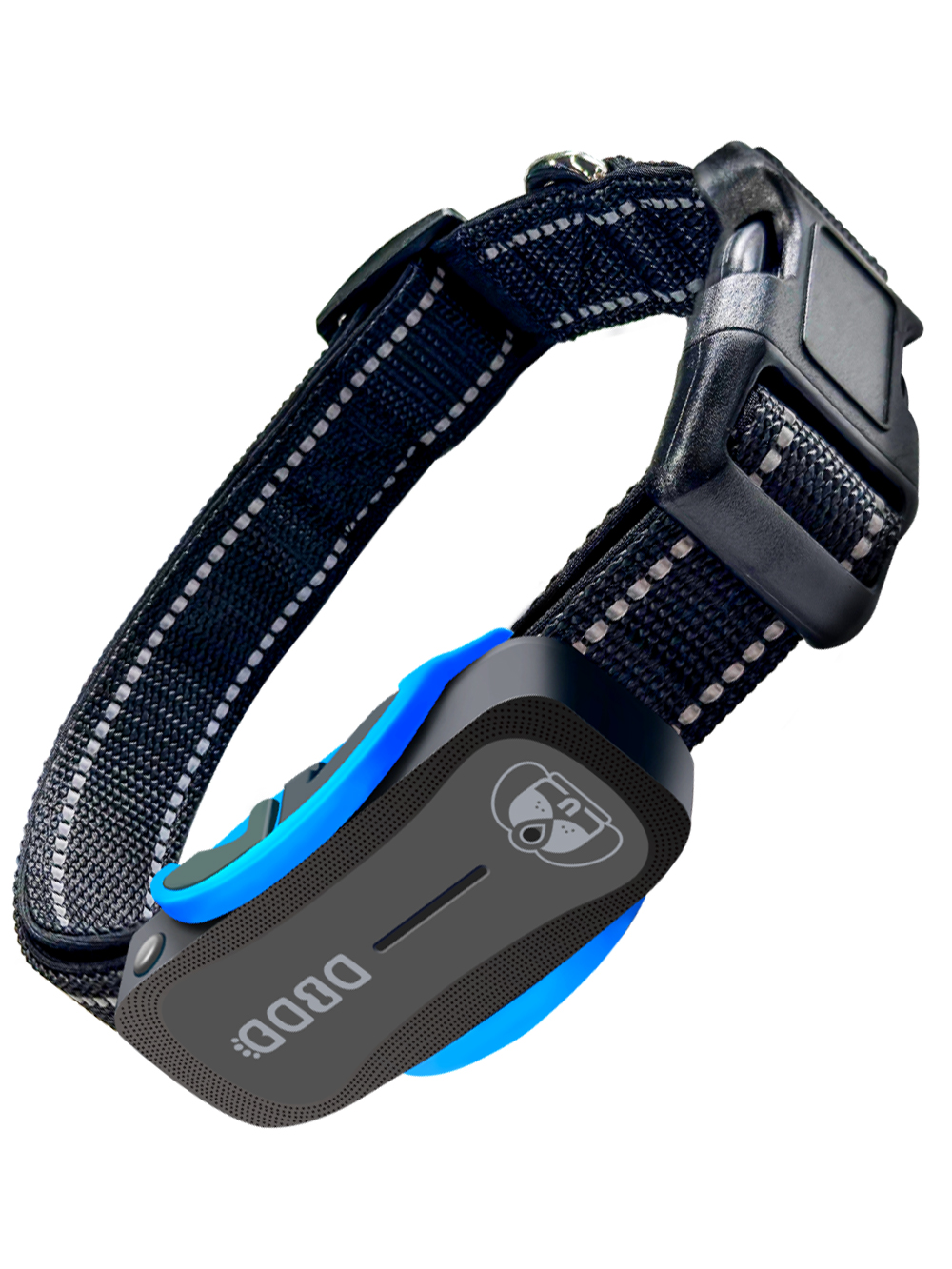
Another challenge lies in the interpretation of dog barking sound. While some barks are relatively straightforward to classify, others can be ambiguous or context-dependent. For example, a bark that indicates excitement might sound similar to one expressing aggression, depending on the circumstances. Machine learning models must be sophisticated enough to account for these nuances and incorporate contextual information to make accurate interpretations.
Privacy concerns also arise with the use of wearable devices that collect audio data. Owners may worry about the security of their pet's information and the potential for misuse. Developers must implement robust encryption and data protection measures to safeguard sensitive data and build trust among users. Transparency in how the data is used and stored is essential to alleviate these concerns.
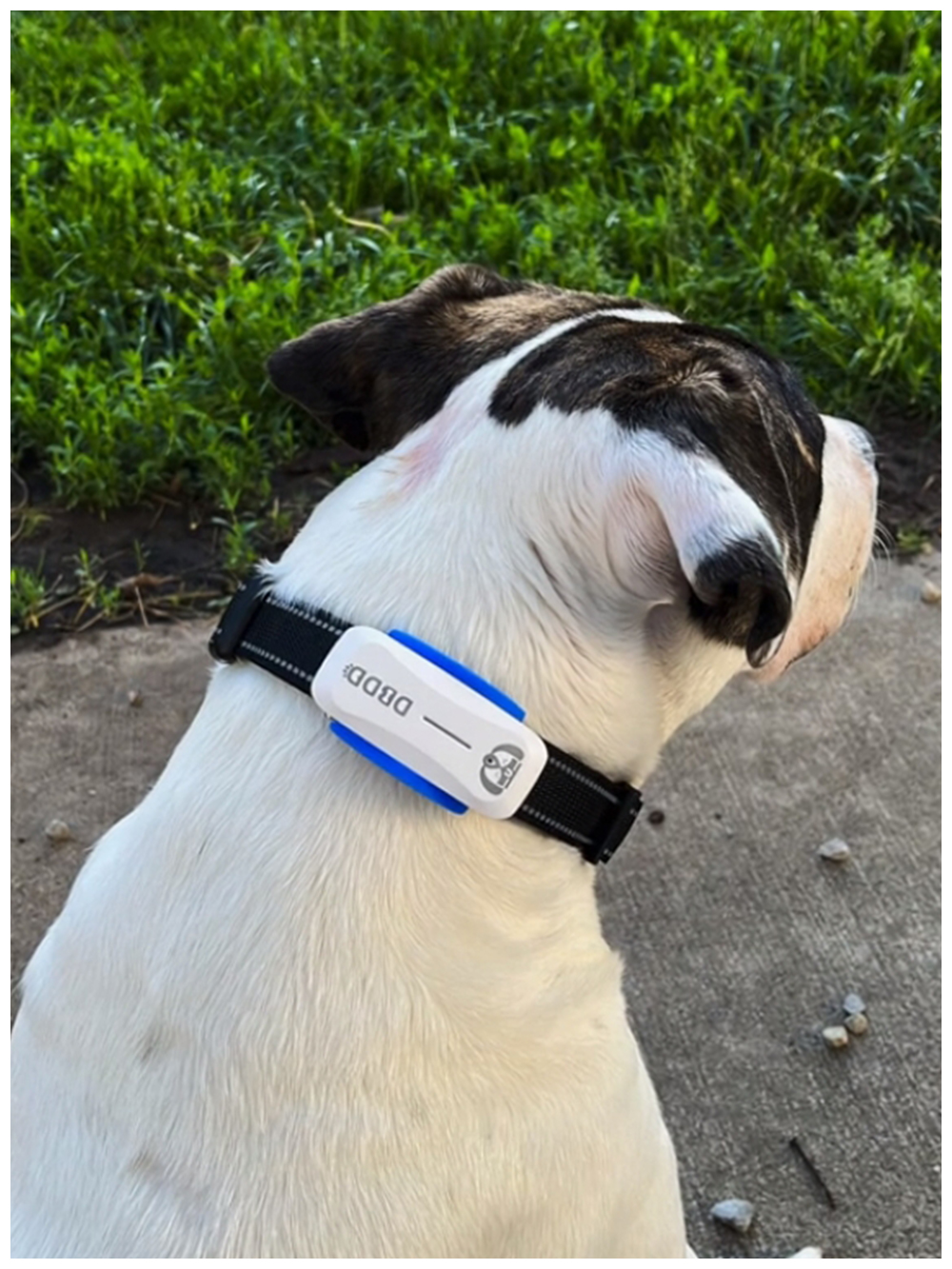
In addition to technical and privacy challenges, there are ethical considerations to take into account. The use of AI in predicting pet behavior raises questions about the extent to which technology should intervene in the natural interactions between humans and animals. Some may argue that excessive reliance on technology could diminish the personal connection between pet owners and their animals. Striking a balance between leveraging AI for practical benefits and preserving the human-animal bond is crucial for the successful integration of these systems into everyday life.
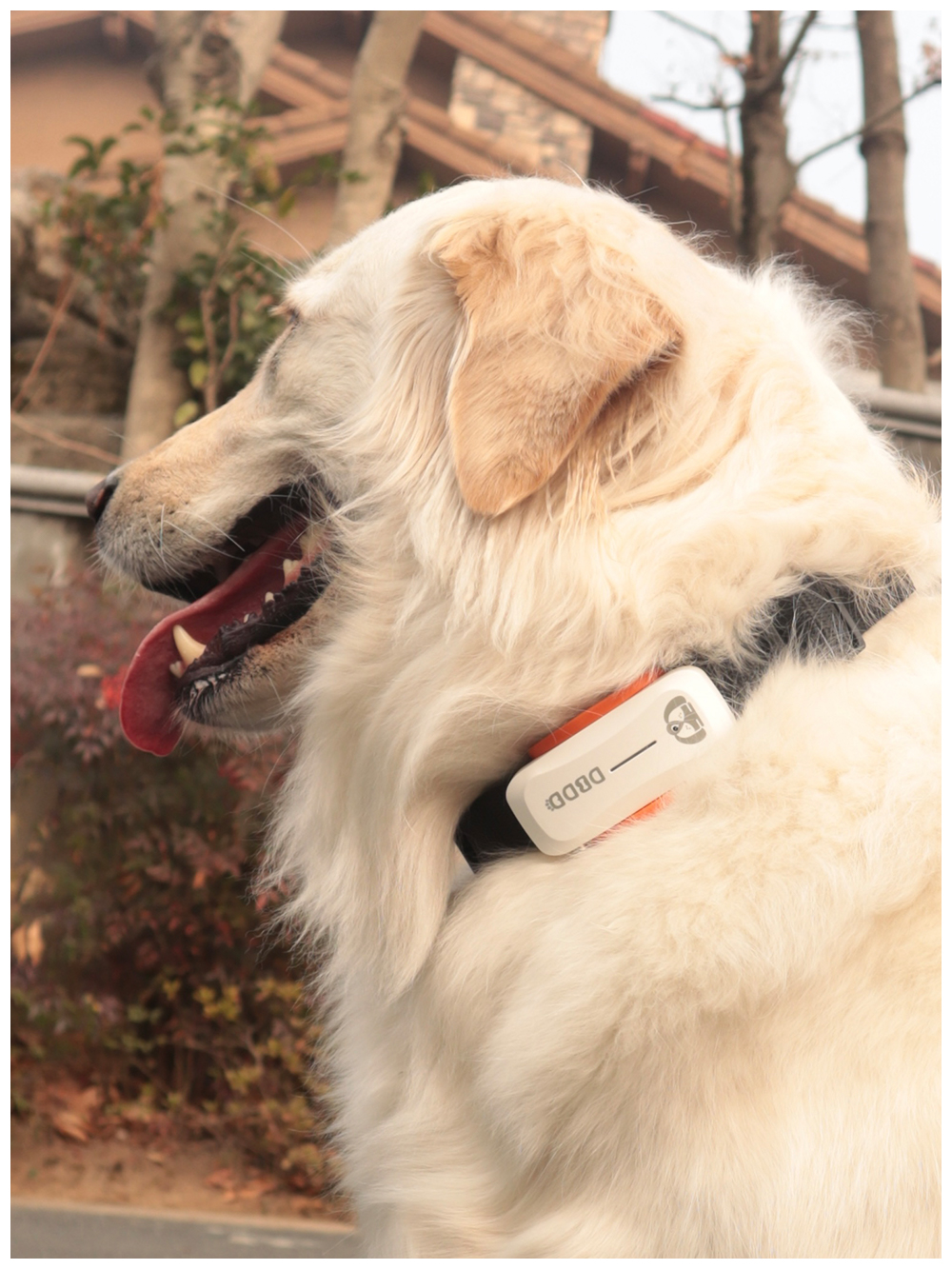
Looking ahead, the potential applications of AI-powered lost pet prediction systems extend beyond simply preventing pets from becoming lost. These systems could also contribute to improving overall pet welfare by providing insights into a dog's emotional and physical well-being. For instance, by analyzing changes in barking patterns, the system might detect signs of illness or discomfort, prompting owners to seek veterinary care sooner. Additionally, the data collected by these systems could be invaluable for research purposes, helping scientists better understand canine communication and behavior.
As the technology continues to evolve, we can expect to see more sophisticated systems capable of handling complex tasks. Future developments might include multi-modal approaches that combine audio analysis with video surveillance or physiological monitoring to create a holistic view of a pet's condition. Furthermore, advancements in natural language processing could enable systems to interpret not only the sounds but also the meanings behind them, bridging the gap between human and animal communication.
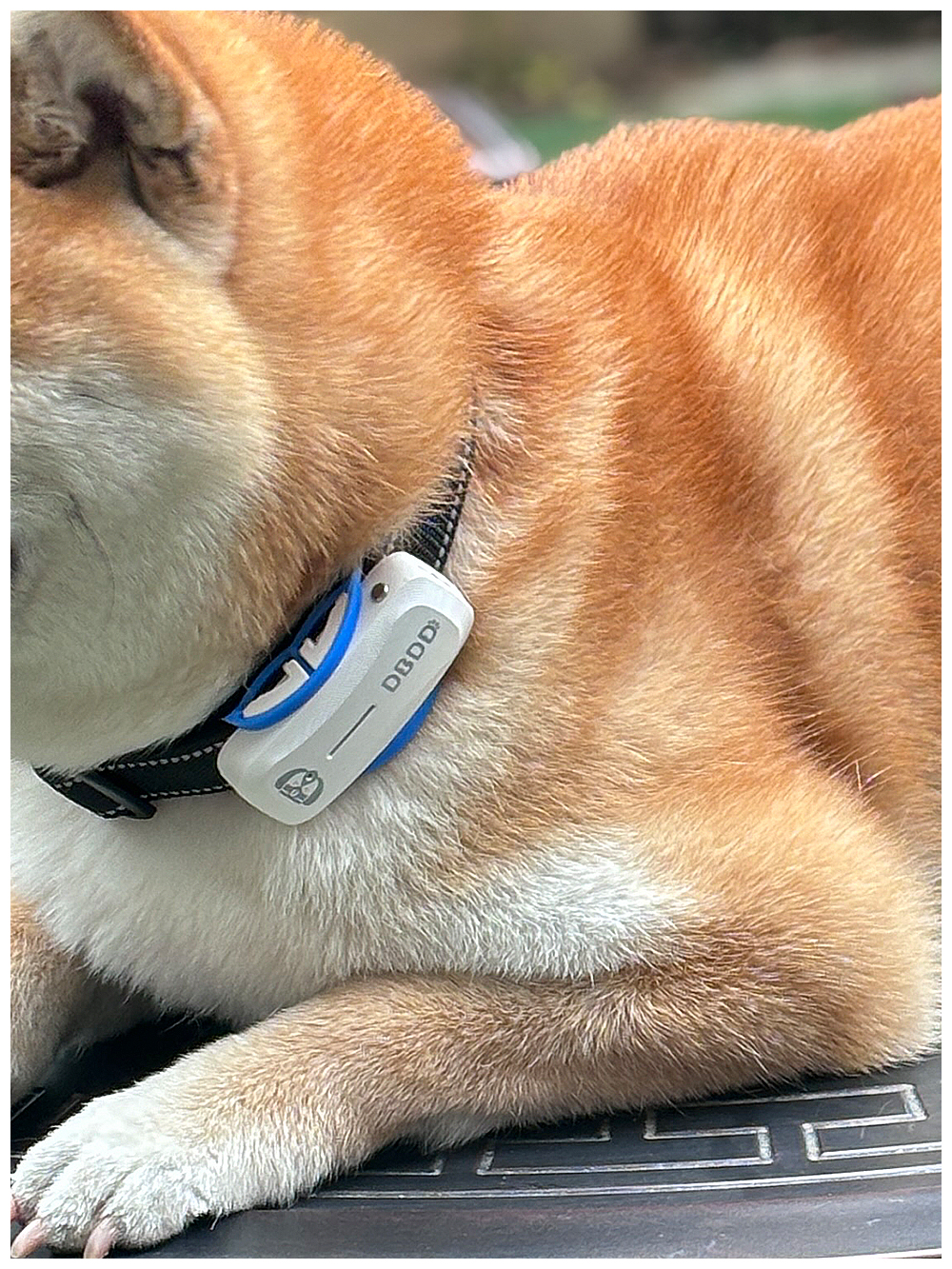
In conclusion, AI-powered lost pet prediction systems represent a promising innovation in the realm of pet care. By harnessing the power of machine learning to analyze dog barking sound, these systems offer a proactive solution to the problem of lost pets. They provide early warnings, facilitate timely interventions, and contribute to a deeper understanding of pet behavior. However, realizing their full potential requires overcoming challenges related to accuracy, privacy, and ethics. With continued advancements and thoughtful implementation, these systems have the potential to revolutionize the way we care for our furry friends, enhancing both their safety and well-being.
Update Time:2025-05-15 07:01:17